Maneuvering your data’s flight path to AI-enabled transformation
Even as enterprises rush to adopt AI, the journey from data to AI isn’t always smooth. Fragmented data, inconsistent standards, and a lack of contextual intelligence frequently hinder the scaling of AI initiatives. In fact, only 20% of AI projects move beyond the pilot stage, and Gartner predicts that by the end of 2025, nearly one-third of Generative AI projects will be abandoned after the proof-of-concept phase.
The challenge isn’t just about building AI; it’s about getting data ready for AI. After all, without good data, there is no AI. Onix has developed Wingspan, a platform built to bridge the gap between data and AI. Wingspan is an agentic AI platform that unifies enterprise knowledge, automates intelligence, and ensures faster AI adoption with smart agents. The platform is designed to help enterprises overcome the most common challenges related to getting data ready for AI adoption.
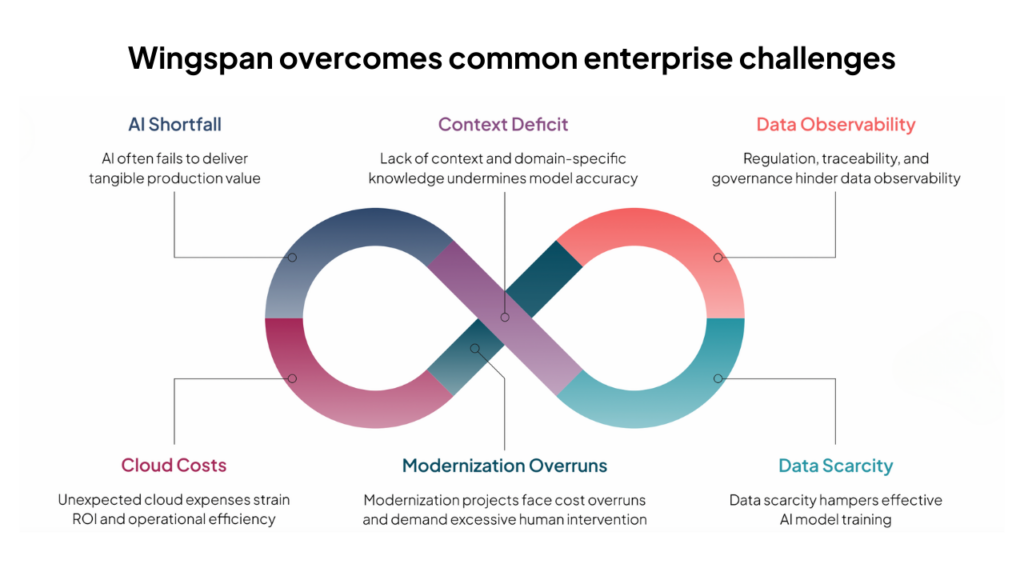
In the following section, we break down six of the most critical enterprise challenges and explain how they impact AI adoption:
-
AI shortfall
90% of GenAI projects will fail to deliver business value in 2025. AI-related failures don’t stem from the technology itself but rather from a lack of business strategy, governance, and poor implementation. Without a realistic ROI strategy, most companies simply rush into AI implementation, thus ending up wasting their investments.
Here are some of the factors causing the failure of AI initiatives in the business domain:
- Lack of structured measurement (or KPIs) for AI investments.
- Lack of a sustainable business strategy.
- Lack of alignment with business objectives and challenges.
- Limited cross-functional collaboration.
How does Onix’s Wingspan solution address this challenge? Wingspan combines the capabilities of its cloud migration and modernization IPs for faster and effective AI adoption. By integrating multiple AI agents, it establishes a comprehensive knowledge graph and business glossary, expediting the deployment of AI initiatives.
Next, let’s examine how AI projects are impacted by data scarcity and fragmentation.
-
Data fragmentation and scarcity
Fragmented data (or silos) makes it difficult for enterprises to access and integrate data for their AI systems. As more AI models depend on a variety of data sources, the problem they face is that enterprise data is stored in varying file formats and structures, which has a direct impact on data aggregation and analysis.
Further, fragmented and siloed data makes it challenging for enterprises to gain a unified view of their data, thus leading to implications such as:
- Process inefficiencies
- Inaccurate reporting
- Data duplication
- Lack of real-time insights
Besides fragmentation, sophisticated AI models now face the problem of data scarcity, which limits the effectiveness of their real-world outputs. Lower the variety of its input datasets, lesser is the preparedness of AI models to address business challenges.
Fragmented data silos and data scarcity make integration hard. However, synthetic data has emerged as a viable alternative for training AI models and overcoming the issues of data scarcity and privacy. Wingspan can deploy the right agent to generate synthetic data that mirrors your production data, ensuring both compliance and model accuracy.
Next, let’s break down how missing context affects AI training.
-
Lack of context awareness in AI model training
Traditional AI models have struggled with the lack of context awareness, effectively limiting their real-world applications. With the lack of domain knowledge and contextual information, AI models produce unreliable outcomes, which can directly impact business decision-making abilities.
With the emergence of Google Gemini, AI models can improve their contextual awareness by integrating text, images, and audio. With these developments, AI models are better equipped to understand the complex nuances of human communications and provide appropriate responses. This led the product engineering team at Onix to develop Wingspan, which builds a deep enterprise knowledge graph to give AI the right context.
Effective data observability is another key aspect that’s crucial for overall data governance and compliance. Let’s explore that aspect next.
-
Data observability
In the wake of growing industry regulations and penalties, effective data observability is critical for enterprises powered by data and AI. Without observability, enterprises can face several “blind spots” in implementing data governance. On the other hand, an observability-only strategy (without data governance) can lead to data security and regulatory problems.
Observability improves data governance by monitoring data quality for issues like missing or inconsistent data. This is important as 85% of enterprises struggle with low-quality data, thus leading to financial losses.
While “traditional” observability tools appear scattered and have limited context, Onix’s Wingspan implements data observability with its embedded Agentic AI capabilities across the entire data lifecycle. Wingspan provides real-time insights into the data flow, thus building an end-to-end knowledge graph – along with complete business context, data lineage mapping, and traceability. Furthermore, Wingspan can proactively detect data anomalies and pattern deviations with its built-in AI capability.
Next, let’s explore another critical challenge, post-migration cost escalation.
-
Cloud costs escalation
A 2024 report found that AI technologies are driving up cloud expenses by 30%. Over 70% of FinOps practitioners rate GenAI-driven cloud spending as “unmanageable.”
To maximize their AI potential, enterprises are modernizing their legacy systems on the cloud. However, without proper cloud optimization, they can easily exceed their allocated budget to migrate to the cloud. 63% of tech executives regard cloud cost optimization as a key factor – even as 6 in every 10 enterprises are exceeding their cloud budget.
Besides the lack of cloud cost optimization, cloud sprawls are also common among fast-growing companies – primarily due to the uncontrolled proliferation of cloud services, resources, and instances. Apart from creating security problems, a cloud sprawl can also cause cost overruns.
Cost escalation can easily impact the enterprise’s ROI from AI projects as well as their operational efficiency. Wingspan helps control cloud sprawls and costs by optimizing resource allocation, ensuring enterprises stay on track with their AI goals without overspending.
Companies adopting the cloud can also face cost overruns during the modernization phase. Let’s examine this impact next.
-
Modernization overruns
Traditional cloud modernization projects demand advanced technical skills and human intervention, often leading to cost escalation and delays. According to KPMG Research, enterprises overspend 35% on average on their cloud resources. Efficient modernization isn’t just about the initial spending, but also about effective resource management to avoid unexpected expenses in the later stages.
With its autonomous agents, Onix’s Wingspan can automate and accelerate the modernization process by 30−70%, as well reduce costs by 20−35%. As a unified platform, Wingspan delivers an integrated approach to the entire modernization process, while reducing dependence on human resources.
How Onix’s Wingspan can address these AI challenges
As mentioned in this press release, Onix is launching Wingspan as the industry’s first agentic AI platform that accelerates the data-to-AI journey and adoption of modern data platforms. Empowered by autonomous AI agents, Wingspan can be the game-changer for enterprises transitioning to modern data platforms and AI applications. By deploying this tool, enterprises can now accelerate modernization by 2-3x – and operationalize their AI systems in the production phase within just 4 weeks.
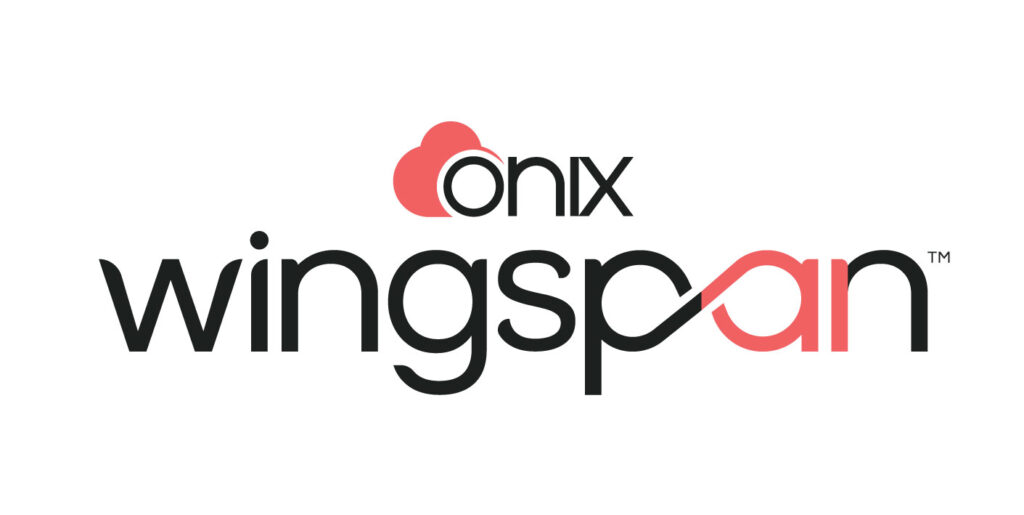
Embedded with the power of Onix’s Birds suite of tools, Wingspan is designed to deliver the following AI-enabled capabilities:
- A unified and centralized view of enterprise data with Eagle’s knowledge graph feature.
- A common and consistent data framework for managing data definitions, thus ensuring clarity and accuracy across the entire organization.
- A centralized platform to create, deploy, manage, and monitor AI-powered agents.
- The development and deployment of customized LLMs for specific business domains, thus enabling relevant AI-powered insights.
- A robust AI security framework to secure AI models, data, and agents, thus ensuring regulatory compliance and risk mitigation.
With its end-to-end compatibility with Google Cloud’s AI products including Google Agentspace, Wingspan is effectively a comprehensive “data to AI” platform that navigates enterprises from raw data to real-world AI solutions.
Conclusion
Onix’s Wingspan can elevate cloud modernization – and accelerate the deployment of AI models with context awareness. As compared to traditional AI tools, this platform leverages the power of AI agents to accelerate your modernization process.
Recognized as the 2025 Google Cloud Partner of the Year for Data & Analytics and Industry Solutions – Telecommunications, Onix is set to reshape AI deployment and cloud transformation with its technology-driven innovations. With the launch of Wingspan, Onix is taking one step ahead in its commitment to deliver AI-ready solutions.
Do you want to learn more about Wingspan? Read more about this innovative solution in our latest press release – or reach out to us today.
Reference links:
5 Reasons Why AI Projects Fail (And How to Avoid Them)
Data fragmentation inhibits AI’s potential.
Will AI Models Ever Understand Context? The New Frontier of Deep Learning in Contextual Awareness
AI Systems and Data Products: Finding Common Ground
Data Scarcity: When Will AI Hit a Wall?
6 Change Management Strategies To Avoid Enterprise AI Adoption Pitfalls